Most marketers know not to chase shiny objects. But if prospects are attracted to them, aren’t they worth investigating? Once upon a time, digital ads were so new and sparkly that companies felt silly using them. See below, the first-ever digital ad, by AT&T.

First digital ad by AT&T
Email marketing was also once a shiny object, roundly criticized as spam. So were coupons, direct mail, and websites. Look at them all now.
Every dominant channel or tactic begins as nothing and typically, those who bet on it early win big. AT&T’s CTR for the ad above was 54 percent. So how do you know where to bet? You let your team chase the occasional shiny object but teach them to rigorously test it with hypothesis-based marketing. That way, every tool, tip, and tactic either proves its worth in the gladiatorial ring of funnel conversions where only the strongly correlated survive, or it falls out of use.
This is how my team occasionally achieves paradigm shifts for our clients. Instead of struggling to boost campaign conversions incrementally, we stumble upon entirely new strategies that boost performance exponentially. This is a lot better than following generalized ‘best practices’ that by the nature of the fact that everyone’s doing them, aren’t.
You can take heroic leaps too. Here’s how.
Testing is where B2B often fails
Everyone today talks about being data-driven but I’d argue that in B2B, most of it is just vanity testing. Most companies rely on features in their MAP to experiment with headlines, copy, images, and notoriously, button colors and stop there. It’s all one-off, isolated, campaign-level testing with limited impact. They aren’t testing larger strategies, like whether to run those campaigns at all or pitting campaigns against one another.
Perhaps they do it because that’s all that most MarTech tools are designed and developed to do. They view the world in terms of campaign objects, and can’t test what they can’t see, and so they don’t encourage strategic-level testing.
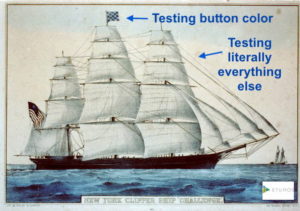
The limited returns of late-stage testing
Few marketing teams step back to test bigger stuff. Like whether they should even send emails at all. Those are the tests with exponential returns because you can apply those lessons across all campaigns, strategies, messaging, and targeting. Rather than a five percent increase on one campaign, it’s a five percent increase in ten different areas.
Strategic-level testing obeys the principle of exponential growth: Results stack upon one another, and companies doing this kind of testing have very different trajectories.
Even when I do see a B2B company running strategic-level tests, many commit a serious error: They don’t have a system for sharing those lessons internally. This prevents them from applying those lessons throughout the business. Even if they do discuss their learnings and share positive and negative results, few templatize that knowledge.
All of this is why at Etumos, we’ve instituted a culture of math-based marketing where we train our team to step back and recognize that we are scientists. There’s a world beyond the campaign object microscope, and it’s our job to document it.
How we get scientific at Etumos
Our five-step system for testing:
1. Prioritize tests based on their expected reward
Identify a weak link in your conversion funnel. If you’re being scientific about your marketing, the conversion funnel is the truest barometer of progress. Everything you test should touch it in some way, and raise one of your conversions. For example, say your team’s conversion from MAL to MQL is five, lower than the industry standard of seven (rough numbers here). What is raising that conversion two points worth to the business? If you do the math, that’s your expected marketing bounty, or the estimated cost or revenue impact from tackling this problem. If you standardize those bounties, you can see which tests have the biggest test payouts. Run the most lucrative ones first.
2. Develop a hypothesis
Look at what customers experience at the conversion stage in question. Are site visitors filling out forms but not returning? Are they getting emails but not responding? Write a hypothesis that can be proven or disproven.
For example:
Hypothesis: If we use a personalization engine that shows industry-specific content to visitors, it’ll increase our MAL to MQL conversion rate two points or more.
3. Assign a time period
At Etumos we usually test for one calendar quarter. Once you know the period, you can determine the expected dollar per stage:
(expected bounty for the time period) x (hypothetical increase in conversion rate)
For example, if MALs normally have a six percent conversion rate and the throughput is 12,000 leads per quarter, an increase of one percent results is a bounty estimate of 1,200 leads/quarter. Multiply this by the expected opportunity value of MALs ($30), and the expected bounty value in revenue is $36,000 per quarter.
This works for anything. You can even determine the overall contribution of marketing to revenue, if you like, by performing a global holdout.
And, we don’t just look at closed-won deals. It’s great to measure those when you can because it’s real money, but this is B2B marketing. Sales cycles are long and the final number of closed-won deals can be small, even at large enterprises. In order to have a respectable sample size, we often need to measure higher up in the funnel where we can work with larger samples.
You won’t have to wait months for deals to progress to measure quality, either. Simply look at how two cohorts convert from MQL to SQL to determine their comparative meeting set rate.
4. Test with a statically significant sample (when possible)
We build our client’s MAP instances for constant testing. We randomly assign a cohort to each new contact that enters the system, and when we run a test, we use those cohorts. Then, we ensure we have (when possible) a statistically valid sample size.
A rule of thumb we use for this is to involve at least 1,000 persons. But in a pinch, you could do with as few as 100.
A few points of order when setting up your tests:
- Use a control group if you need to establish a firm cause and effect. If, on the other hand, you’re only observing a new channel and already know your standard conversion, this may be unnecessary.
- Only test one (or more) things at a time if you have a random sampling. Don’t test two elements per cohort (for example, a different channel and different format) unless you’re certain the samples are random and the tests are discrete.
- Don’t interrupt your test. A study by Wharton found that 59% of marketers inadvertently conduct what’s known as p-hacking, where they end tests early when they get the result they want. This invalidates the test because results could just as easily swing the other way before it’s complete.
- Where to keep the data? Stay organized across teams and standardize where you save and share information. At Etumos, our MOPs team keeps the data inside the MAP and exports it to a statistical analysis program or at the least, Excel or Google Sheets when needed. They record their lessons in a shared Google Doc.
5. Evaluate results, build knowledge
Did your results prove or disprove the hypothesis? Did you get the seven percent conversions you wanted? Share that knowledge with the team. It’s vital that those lessons, positive, negative, or neutral (no correlation) are spread so that others don’t go testing whether content personalization does the trick, only to spend more resources repeating that test.
The Etumos team records their lessons in a shared doc. Once per month, we review them as a group and codify the most useful ones into rules, or revise existing rules. At a bigger organization, you might even ask someone on the team to share learning updates in a department newsletter.
By tracking lessons over time, we’re able to identify the difference between strategic best practices, which are fundamental and unchanging, and tactical ones such as send times, guilt-tripping pop-ups, and “looks like you got eaten by a hippo” emails that are only effective because of their novelty or relative scarcity. Hippo emails had their day in the early 2010s, but are now hopelessly ineffective.
The future of hypothesis-based marketing
What works for us can work for others and I like to imagine a near future where marketing case studies are so rigorous they’re peer-reviewable. Marketing leaders and their teams could collect empirical proofs and a library of replicable lessons. These lessons would build upon prior lessons and successes would beget more successes. I think hypothesis-based marketing is how we get there.
It makes marketing a mathematically respectable profession and gets CMOs speaking their CEO’s ‘business value’ language. It changes that conversation from skepticism to encouragement. From: ‘Why are we spending money on shiny objects?’ To: ‘What have we learned from our tests and what can it tell us about the future of our business?’
Appendix 1: Where can I begin?
Try a global holdout to measure how much your nurture programs contribute to your conversion rate and funnel overall. It’s expensive to handicap a group of leads, sending them to a purposefully less effective group, so make the group as small as possible while still being statistically significant, but it can be invaluable to know whether your primary email activities do what you think they do. Many companies are in for a big surprise.
Another good first foray is to conduct a funnel gap analysis for your core revenue model, determine the greats conversion rate opportunity compared to industry standards, and then test hypotheses to increase that conversion rate.
Appendix 2: Some considerations
Every rule has its exceptions, but the more I’ve tried to run our marketing by math rather than opinion, the more I’ve found that nearly everything is testable. And if tests increase conversions, tests make our clients money.
Even intangibles like billboards can be tested. All data is an abstraction, so testing for brand affinity and preference isn’t so different than testing email opens if you have some discrete conversion action you can measure. (Advertising agencies have been doing this since at least the 60s.) Simply create a controlled test where you’re measuring the aggregate lift in conversion rate (and corresponding lower cost-per-qualified-lead) based on a cohort of “in the geographic area that’s likely to see this billboard” versus a controlled cohort without any billboards. This can reveal the incremental dollar value of a non-digital channel.
Finally, pick your battles. A large part of smart hypothesis-based marketing is knowing when to ask your team to invest the 4-5 hours to set up a test or not. Pick the biggest impact areas and bounties, and use the conversion funnel as your guide.